Method for Reducing the Dimension of Training Sets at Constructing Neuromorphic Fault Dictionary for Analog Integrated Circuits |
|
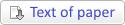 |
|
|
Authors |
| Mosin S.G. |
Date of publication |
| 2018 |
DOI |
| 10.31114/2078-7707-2018-2-59-63 |
|
Abstract |
| Machine learning methods are actively used for the construction of neuromorphic fault dictionaries (NFD), which provide diagnostics of faults in analog and mixed-signal integrated circuits in the associative mode. Many problems of a neural network training associated with a large amount of raw data can be solved by reducing the dimension of training sets and using only significant char-acteristics for training purpose.
Entropy-based method is proposed for selecting the essential characteristics of a training set, as well as a cor-responding algorithm is developed.
The study of the proposed method is performed for the benchmark circuit of the Sallen-Key analog filter. The results of experimental studies demonstrate the high efficiency of the proposed method.
The application of the proposed method has provided a reduction in the dimension of the training set by selecting the sufficient coefficients in 7.8 times and reducing the training time by 192 times, while demonstrating a high level of fault coverage. The resulting NFD provides coverage up to 100% of individual faults and up to 99.7% of the overall fault coverage for the filter.
The proposed method can be integrated into the design-for-testability flow for analog and mixed-signal ICs. |
Keywords |
| fault diagnostics, neuromorphic fault dictionary, analog integrated circuits, entropy, machine learning, design automation. |
Library reference |
| Mosin S.G. Method for Reducing the Dimension of Training Sets at Constructing Neuromorphic Fault Dictionary for Analog Integrated Circuits // Problems of Perspective Micro- and Nanoelectronic Systems Development - 2018. Issue 2. P. 59-63. doi:10.31114/2078-7707-2018-2-59-63 |
URL of paper |
| http://www.mes-conference.ru/data/year2018/pdf/D010.pdf |