Home
Authors Papers Year of conference Themes Organizations To MES conference
Neural Networks Models based on the tensor-matrix theory |
|
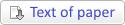 |
|
|
Authors |
| Slyusar V.I. |
Date of publication |
| 2021 |
DOI |
| 10.31114/2078-7707-2021-2-23-28 |
|
Abstract |
| The versions of the mathematical formalization of the neural hypernetworks based on the family of penetrating face products of matrices and tensors expanded to the block matrices are considered. As an example, the matrix A in the penetrating face product of matrix A and block matrix B can be considered as a picture pixels matrix on the input of a neural network. In this case, every block of matrix B corresponds to a block of weight coefficients for a few neurons in one layer of the neural network. Further steps of data processing in the considered neural network can be varied different depending on the structure and type of layers of neural network. In the case of convolutional neural networks the result of penetrating face products of matrices A and B has to multiplied by a vector of one’s 1. This multiplication can produce a scalar, a vector-row, a vector, or a matrix. The result of such multiplication can be used as argument of an activation function. For the data processing in hierarchies of neural hypernetworks clusters, the generalized face-splitting products of matrices and block version of these multiplications can be used. The operation of a block penetrating Kronecker product of matrices has been introduced to simulate the input layer of a neural hypernetwork which process multiple video streams from several video cameras in different spectral ranges in parallel by a set of several neural networks. |
Keywords |
| matrix, tensor, tensor-matrix theory, neural network, hypernetwork, activation function, penetrating face multiplication, generalized face-splitting product, block generalized face-splitting product, block penetrating Kronecker product. |
Library reference |
| Slyusar V.I. Neural Networks Models based on the tensor-matrix theory // Problems of Perspective Micro- and Nanoelectronic Systems Development - 2021. Issue 2. P. 23-28. doi:10.31114/2078-7707-2021-2-23-28 |
URL of paper |
| http://www.mes-conference.ru/data/year2021/pdf/D025.pdf |
|
|